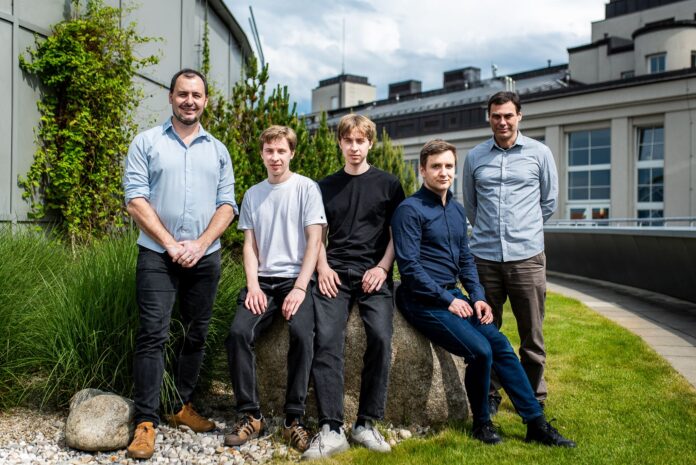
This year’s winner of the Neuron Award for young promising scientists, Dr. Tomáš Pluskal from the Institute of Organic Chemistry and Biochemistry of the Czech Academy of Sciences, together with his student Roman Bushuiev and colleagues from the Czech Institute of Informatics, Robotics and Cybernetics at the Czech Technical University (CIIRC CTU), Dr. Josef Šivic and Anton Bushuiev, have developed a machine learning model called DreaMS, which significantly accelerates the analysis of previously unknown molecules. The study was published in the influential scientific journal Nature Biotechnology.
Nature is full of chemicals that have yet to be discovered. It is believed that the vast majority of natural molecules remain unknown. Describing them could pave the way to new drugs, more environmentally friendly pesticides, a deeper understanding of biological processes, or more advanced research into life in the universe.
Each substance has a unique pattern, similar to a human fingerprint, called a mass spectrum, which can be captured using a method known as mass spectrometry. Although this approach generates large quantities of data, interpreting it and uncovering exact molecular structures is extremely difficult. The resulting datasets often appear as vast tables of numbers with no obvious meaning.
To unravel the mystery of unknown molecules, the team from IOCB and CIIRC CTU turned to artificial intelligence. Much like large language models such as ChatGPT learn to understand language without knowing the meaning of words in advance, the DreaMS model attempts to interpret mass spectra without prior knowledge of their chemical structures. “ChatGPT can infer the meaning of words and the connections between them from large volumes of text, and the DreaMS neural network, using self-supervised machine learning, learns to recognize what molecular structures are hidden within spectra. It draws on data from millions of examples,” explains Josef Šivic.
“The DreaMS model was trained on tens of millions of spectra from diverse organisms and environments – plants, microbes, food, tissue, and soil samples. Thanks to this, it can uncover hidden similarities between spectra that, at first glance, seem unrelated,” says Tomáš Pluskal. The result is an interconnected network that helps navigate the vast body of chemical data. This network, which can be imagined as an internet of mass spectra, has been named the DreaMS Atlas. Each spectrum is like a website linked to others. On this “internet of spectra”, users can search, explore discovered connections, and ask new questions – for example: What do pesticides, food, and human skin have in common? DreaMS uncovered unexpected chemical similarities between them and hypothesized that certain pesticides may be linked to autoimmune diseases such as psoriasis.
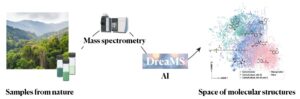
In addition to connecting spectra from different studies, DreaMS can also be used for various practical tasks – for instance, to estimate how many specific fragments a molecule contains or whether it includes particular chemical elements. “We were especially surprised that the model learned to detect fluorine,” says Roman Bushuiev. “Fluorine is present in about one-third of all drugs and agrochemicals, but we were previously unable to reliably detect it from the mass spectrum. After pretraining DreaMS on millions of spectra, we fine-tuned it with a few thousand examples of fluorine-containing molecules – and suddenly it worked.”
The researchers are now working on the next step: teaching the model to predict entire molecular structures. If successful, it could fundamentally transform our understanding of chemical diversity – whether on planet Earth or beyond.
Original Article: R. Bushuiev, A. Bushuiev, R. Samusevich, C. Brungs, J. Sivic and T. Pluskal, Self-supervised learning of molecular representations from millions of tandem mass spectra using DreaMS Nature Biotechnology (2025)
https://doi.org/10.1038/s41587-025-02663-3
Josef Šivic also comments on the topic in a report on Český rozhlas – Věda Plus – you can listen to the whole interview HERE.